Unlocking Potential with Image Annotation Tools in Machine Learning
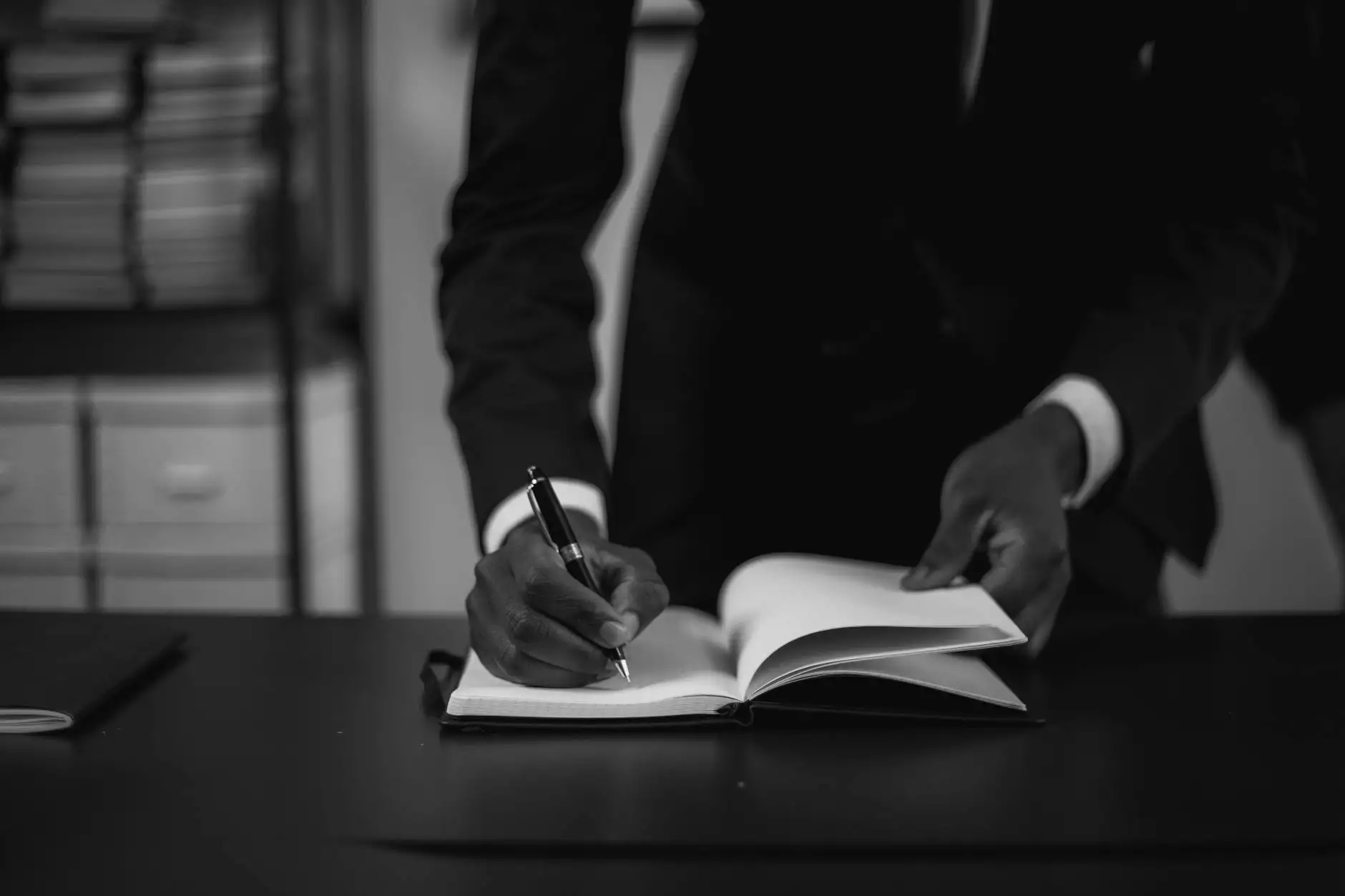
In today’s rapidly evolving technological landscape, image annotation tools have emerged as a vital component that fuels the development of machine learning models. This article explores the significant role these tools play in machine learning, the different types of annotation techniques available, and how businesses can leverage them through effective software development strategies.
Understanding Image Annotation
Image annotation refers to the process of labeling images to create datasets that train algorithms to recognize patterns and make predictions. These annotated datasets are crucial for training deep learning models, enabling computers to understand and interpret visual data, as humans do.
The Importance of Image Annotation in Machine Learning
As machine learning continues to permeate various industries, the importance of high-quality annotated datasets cannot be overstated. The following points illustrate the significance of image annotation:
- Enhanced Model Accuracy: Properly annotated images improve the accuracy of machine learning models by providing clear examples of what to learn from.
- Data Diversity: Comprehensive annotation across a variety of images can enhance a model's ability to generalize from new data.
- Automated Learning: Annotation allows models to learn in an unsupervised or supervised manner, drastically reducing the time and cost involved in manual data labeling.
- Sector-Specific Applications: Different industries, like healthcare, automotive, and retail, can greatly benefit from customized image annotation to meet specific needs.
Types of Image Annotation Techniques
There are several effective image annotation techniques used in machine learning, each serving unique purposes. Let’s delve into the most commonly used techniques:
1. Bounding Box Annotation
This technique involves drawing rectangles around objects within an image. It is primarily used in object detection tasks to delimit areas of interest, making it useful for applications such as self-driving cars where identifying objects like pedestrians and vehicles is critical.
2. Semantic Segmentation
Semantic segmentation provides a more detailed view by classifying all pixels in an image into categories. This allows models to understand context and object relationships, making it invaluable for applications like autonomous vehicles or medical imaging.
3. Instance Segmentation
This approach combines the effectiveness of bounding boxes and semantic segmentation, distinguishing between object instances within the same class (e.g., differentiating between two cars in an image). This is significant for tasks requiring high precision in detection.
4. Landmark Annotation
In this method, specific points on objects are annotated to provide a reference for their location. This technique is essential in facial recognition technologies, where identifying key facial features can improve accuracy.
5. Polygon Annotation
Polygon annotation allows for the outlining of irregularly shaped objects. This technique is beneficial in applications where precision is essential, such as in agricultural technology for identifying crop boundaries.
How Image Annotation Tools Facilitate Machine Learning
The advancements in technology have led to the development of sophisticated image annotation tools that simplify the annotation process, ensuring that businesses can create high-quality datasets efficiently. Here’s how these tools contribute to the success of machine learning initiatives:
User-Friendly Interfaces
Modern annotation tools come equipped with intuitive user interfaces that allow users to annotate images quickly, significantly reducing the time required for dataset preparation. This user-friendliness enables teams, even those with limited technical expertise, to participate in the annotation process.
Automation and AI Assistance
Some annotation tools incorporate AI and machine learning algorithms to automate parts of the annotation process. This feature helps minimize human error while sparing time and resources, allowing teams to focus on higher-order tasks.
Collaboration and Cloud Integration
The best image annotation tools support collaboration by enabling multiple users to work on annotations simultaneously. Cloud integration further facilitates easy access to annotated datasets and enables team members to collaborate from different locations.
Quality Control Mechanisms
Effective tools include quality control features that review annotations for consistency and accuracy. These features ensure that the final datasets meet the high standards necessary for successful model training.
Case Studies: Success Stories of Machine Learning and Image Annotation
Several industries have recently begun implementing image annotation tools to leverage machine learning, resulting in transformative outcomes. Let’s explore a few case studies that exemplify this trend:
1. Healthcare Imaging
In the healthcare sector, image annotation has been utilized to develop models capable of detecting diseases in medical images, such as X-rays and MRI scans. For example, by annotating thousands of medical images, AI systems can learn to identify early signs of cancer, leading to timely and potentially life-saving treatments.
2. Autonomous Vehicles
Autonomous driving technology relies heavily on image annotation for training systems that detect pedestrians, road signs, and other vehicles. Companies that have deployed sophisticated annotation tools have dramatically reduced the time taken to develop and enhance their autonomous systems, boosting reliability and safety in real-world driving scenarios.
3. Retail and E-Commerce
In the retail space, machine learning models that rely on image annotation help in visual search technologies. By annotating product images, retailers can enhance their online shopping experience, allowing customers to find products based on images similar to those they upload.
Choosing the Right Image Annotation Tool
With many options available, businesses must choose the right image annotation tool tailored to their specific needs. Here are essential factors to consider:
- Ease of Use: The tool should have an intuitive interface that minimizes the learning curve.
- Customization: Look for tools that allow customization based on specific project requirements.
- Scalability: Choose a solution that can grow with your business needs, accommodating increasing data volumes.
- Integration: Ensure that the tool integrates seamlessly with your existing workflows and machine learning frameworks.
- Support and Training: Assess the level of customer support and training provided by the tool's developers.
The Future of Image Annotation in Machine Learning
As machine learning continues to evolve, so does the role of image annotation. Emerging trends include:
- Increased Automation: Expect to see advancements in automated annotation techniques using AI, further reducing human involvement in data labeling.
- Integration of Augmented Reality (AR): AR technology may enhance the annotation experience, providing real-time context and interactive tools.
- Expansion into New Sectors: New applications for image annotation in sectors such as sports analytics and environmental monitoring are on the horizon.
Conclusion
The role of image annotation tools in machine learning is indispensable. As businesses strive to harness the power of AI and ML, the importance of quality datasets cannot be underestimated. By investing in effective annotation strategies and tools, companies can unlock new potential, drive innovation, and achieve substantial competitive advantages. The future is bright for organizations embracing the synergy between image annotation and machine learning.
Explore Keymakr for Your Annotation Needs
At Keymakr, we provide top-notch image annotation tools tailored to enhance your machine learning endeavors. Contact us today to learn how our solutions can help elevate your business to new heights.
image annotation tool machine learning