Maximizing Efficiency with Labelling Tool Machine Learning
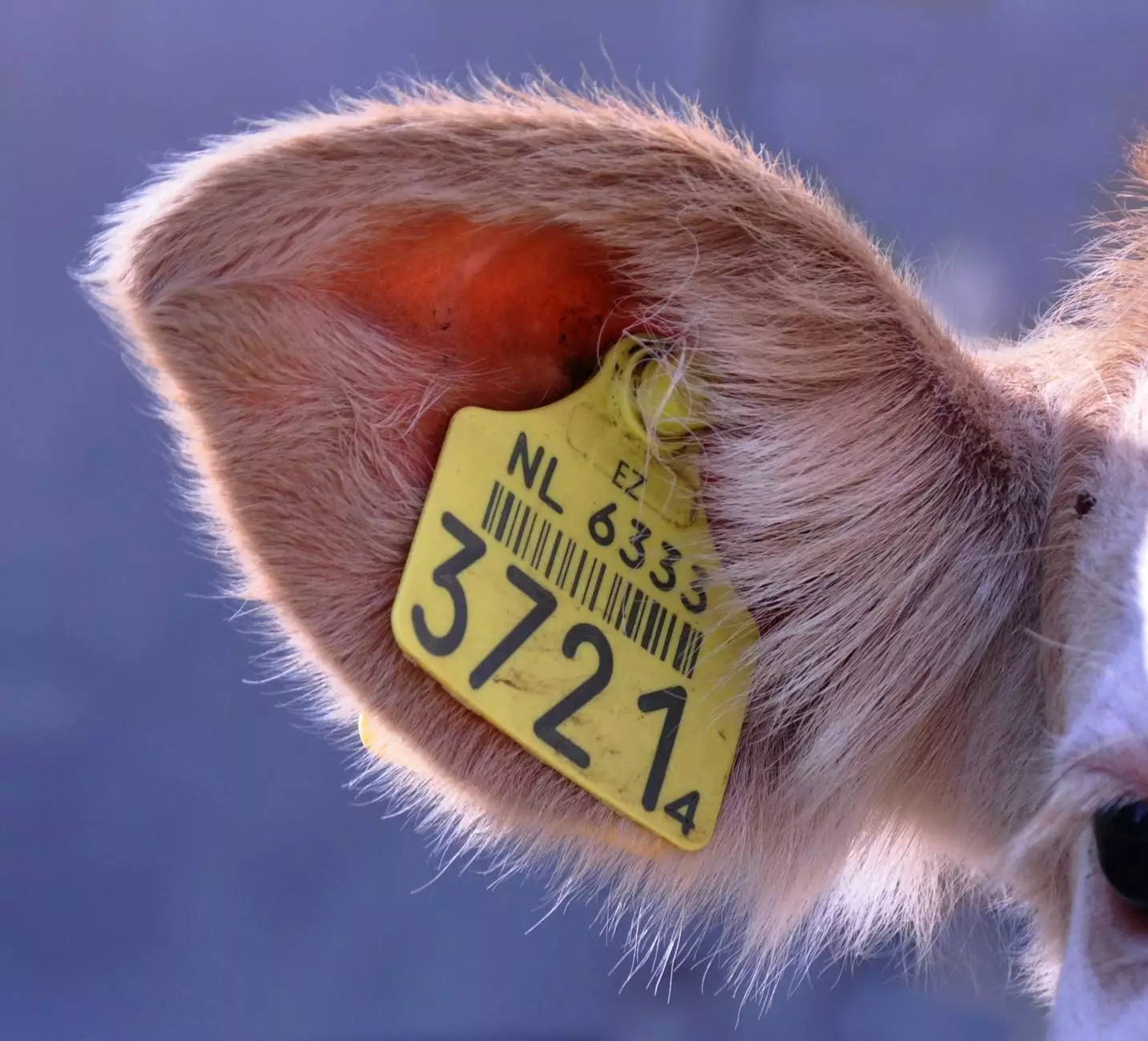
In today’s data-driven world, businesses are continuously seeking ways to enhance their data annotation processes. Implementing a labelling tool machine learning system can significantly improve productivity and accuracy in various fields, including AI development, image recognition, and natural language processing. This article explores the benefits, features, and implementation processes of using a labelling tool powered by machine learning technology.
Understanding Labelling Tools in Machine Learning
A labelling tool is an essential component of the machine learning ecosystem. It facilitates the process of identifying and tagging dataset features, enabling machines to learn from labeled examples. In a typical workflow, data needs to be annotated accurately for the model to understand and predict outcomes effectively.
The Importance of Data Annotation
Data annotation is the process of tagging data with relevant information to train machine learning models. Well-annotated data serves as the foundation for successful machine learning outcomes. Key considerations include:
- Accuracy: Ensuring labeled data is correct to improve model performance.
- Consistency: Using uniform criteria across datasets to avoid bias.
- Scalability: Efficiently managing large volumes of data annotation without compromising quality.
Benefits of Using Labelling Tool Machine Learning
Integrating a labelling tool with machine learning capabilities offers numerous advantages for businesses looking to optimize their data processes:
1. Enhanced Accuracy
Machine learning algorithms can analyze vast datasets more accurately than manual processes. They learn from previous annotations and can pinpoint errors, leading to improved labeling accuracy over time. Features such as auto-suggestions and error detection help users annotate data with precision.
2. Increased Efficiency
Implementing automated labelling tools can reduce the time it takes to annotate data significantly. By leveraging machine learning algorithms, businesses can process large datasets in minutes rather than hours or days. This efficiency allows teams to focus on higher-level tasks rather than manual data entry.
3. Cost-Effectiveness
While there may be initial costs associated with deploying a labelling tool, the long-term savings can be substantial. Reduced labor costs, faster project turnaround times, and improved model performance all contribute to a better return on investment.
4. Scalability for Growing Datasets
As businesses expand, so do their datasets. Traditional data annotation methods may struggle to keep pace, leading to bottlenecks. Machine learning labelling tools can scale operations, adjusting to the volume of incoming data without sacrificing quality.
5. Customization and Flexibility
Many labelling tools offer customizable features that allow businesses to tailor the annotation process to their specific needs. Whether it’s setting up different tagging criteria or adjusting workflows, organizations can create a system that best fits their requirements.
Key Features of a Labelling Tool for Machine Learning
Choosing the right labelling tool machine learning involves understanding the critical features that contribute to efficient data annotation:
1. User-Friendly Interface
A clear and intuitive interface allows users to navigate the labelling tool easily. A user-friendly design minimizes the learning curve, making it accessible for all team members, regardless of their technical expertise.
2. Support for Various Data Types
Look for tools that support different types of data, including text, audio, images, and video. This versatility is particularly important for organizations working across multiple domains or projects.
3. Integration with Other Tools
Integration capabilities allow labelling tools to work seamlessly with existing business systems, such as data management platforms or machine learning frameworks. The ability to integrate with tools like keylabs.ai enhances workflow efficiency.
4. Quality Control Mechanisms
To ensure data integrity, effective labelling tools incorporate quality assurance features. This can include multi-user tagging, review processes, and error reporting functionalities that enable teams to maintain high standards throughout the annotation process.
Implementing Labelling Tools in Your Business
Successful implementation of a labelling tool for machine learning requires careful planning and strategy. Here are essential steps to guide you:
1. Assess Your Needs
Begin by evaluating your existing data workflows and identifying areas for improvement. Determine the volume of data you need to annotate and what types of annotations are required for your specific machine learning projects.
2. Choose the Right Tool
Research various labelling tool machine learning options available in the market. Consider factors like scalability, user reviews, and feature sets. Opt for a tool that aligns with your budget and long-term objectives.
3. Train Your Team
Before rolling out the tool, ensure that your team is adequately trained on its functionalities. Conduct workshops to familiarize the staff with the interface, features, and best practices for data annotation.
4. Monitor Progress
Once implemented, continuously monitor the data annotation process. Use metrics to assess the efficiency and accuracy of the labelled data. Regularly reviewing these metrics will help identify bottlenecks or areas that require additional training or support.
Real-World Applications of Labelling Tool Machine Learning
Machine learning labelling tools have transformative potential across various industries. Below are a few significant applications:
1. Autonomous Vehicles
The automotive industry relies heavily on annotated data to train models for autonomous driving systems. Labelling tools help tag images of road signs, pedestrians, and vehicles, providing critical data for improving road safety technologies.
2. Healthcare
In healthcare, machine learning algorithms analyze medical images and patient data to assist in diagnostics and treatment recommendations. Labelling tools ensure accurate identification of anomalies within medical imaging datasets, thus enhancing patient outcomes.
3. E-commerce
E-commerce platforms utilize machine learning to enhance product recommendations and search algorithms. Labelling tools help classify products accurately, improving searchability and customer experience.
4. Natural Language Processing
In the field of natural language processing (NLP), annotated text data is fundamental for training language models. Tools that label contexts, sentiments, and intents help engines like chatbots become more effective in understanding user queries.
Conclusion
In conclusion, the adoption of a labelling tool machine learning solution can significantly enhance the efficiency and effectiveness of data annotation processes across various industries. By improving accuracy, scalability, and cost-effectiveness, organizations can harness machine learning’s true potential. For businesses looking to stay ahead of the competition, investing in a robust data annotation platform, such as those provided by keylabs.ai, is a crucial step towards achieving better operational outcomes. Embrace the power of labelling tools today, and prepare your data for a smarter tomorrow!